About
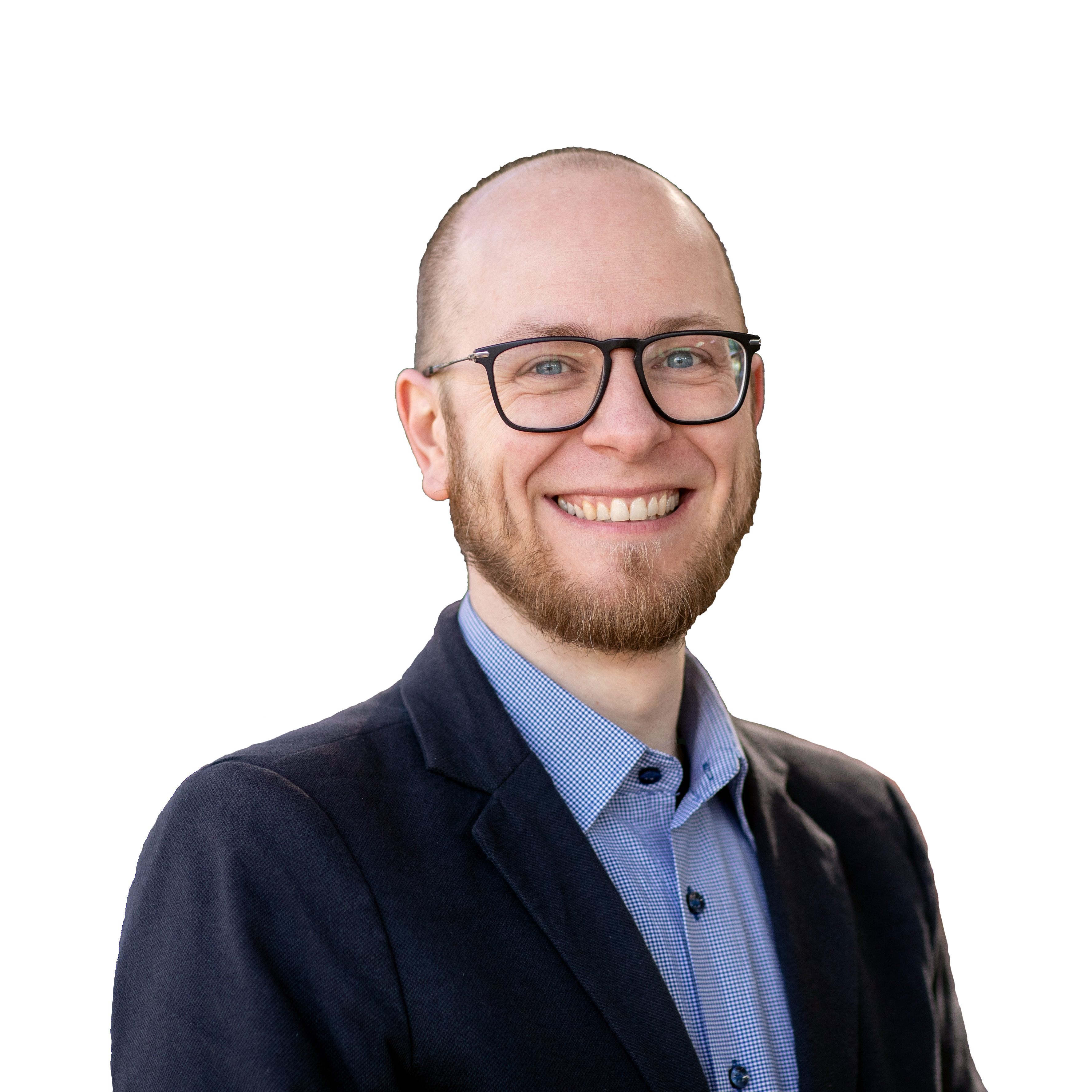
Jakub M. Tomczak
- CV: [PDF]
- Website: jmtomczak.github.io
- Email: jmk.tomczak
gmail.com
- City: Amsterdam, the Netherlands
A Group Leader & Principal Scientist/Research Manager with 15+ years of experience in machine learning, deep learning, and Generative AI. Proven track of leading research projects (academic: 49 AI MSc&PhD, industrial: 7 Computer Vision, 5 LLMs, 2 Foundation Models), carrying out cutting-edge research (2 patents & 1 application, 20+ conference papers: NeurIPS, ICML, ICLR, AISTATS, UAI, CVPR, ICCV, 25+ journal papers), and securing funds (2,150,000 EUR). Experienced in and enjoying managing people (3y in the industry, 8y in academia). Effective team leader encouraging initiative & independence, and facilitating cross-functional collaboration. Well-recognized in the AI community (a PC of NeurIPS 2024, the author of a book on GenAI). The Founder and Director of Amsterdam AI Solutions.
Facts
Years of Experience
Supervised projects
Top publications
Funding gathered (EUR)
Additional Information
Presentations
My presentations at conferences, workshops, for academic and industrial research groups, summer schools
DeeBMed
My project that was carried out from 2016/10/1 to 2018/09/30 within the Marie Skłodowska-Curie Action (Individual Fellowship), financed by the European Comission.
My Book
My book on Generative Artificial Intelligence. This is the first comprehensive book on deep generative modeling explaining theory behind Diffusion-based models, Variational Auto-Encoders, GANs, autoregressive models, flow-based models, and energy-based models. Moreover, each chapter is associated with code snippets presenting how the beforementioned models could be implemented in PyTorch.
Resume
Education
Ph.D. in Computer Science/Machine Learning (with honors)
Mar 2013
Wroclaw University of Technology, Poland
Title: Incremental Knowledge Extraction from Data for Non-Stationary Objects
Supervisor: Prof. Jerzy Swiatek
M.Sc. in Computer Science
Dec 2009
Blekinge Institute of Technology, Sweden
M.Sc. in Computer Science (the best thesis in Poland)
Jul 2009
Wroclaw University of Technology, Poland
Work Experience
Group Leader & Associate Professor, Generative AI
Feb 2023 - Present
Eindhoven University of Technology, Eindhoven, the Netherlands
Founder & Director, Generative AI
Oct 2022 - Present
Amsterdam AI Solutions, Amsterdam, the Netherlands
Principal Scientist, Generative AI for Drug Discovery
Mar 2022 - Present
NatInLab, Amsterdam, the Netherlands
Assistant Professor, Generative AI
Nov 2019 - Feb 2023
Vrije Unviersiteit Amsterdam, the Netherlands
Deep Learning Researcher (Staff Engineer)
Oct 2018 - Dec 2019
Qualcomm AI Research, Amsterdam, the Netherlands
Postdoc (Marie Sklodowska-Curie Individual Fellow), Generative AI
Oct 2016 - Sept 2018
Universiteit van Amsterdam, the Netherlands
Senior Scientist, AI for Drug Discovery
Feb 2016 - Jun 2016
INDATA SA, Wroclaw. Poland
Assistant Professor, Machine Learning
Oct 2014 - Sept 2016
Wroclaw University of Technology. Poland
Postdoc, Machine Learning
Oct 2012 - Sept 2014
Wroclaw University of Technology. Poland
Ph.D. student / Research assistant, Machine Learning
Jun 2009 - Sept 2012
Wroclaw University of Technology. Poland
Publications
Book
J.M. Tomczak, ''Deep Generative Modeling'', Springer, Cham, 2022. [More]
Full list of papers
Please check my Google Scholar
BLOG
- All
- Intro
- ARMs
- Flows
- VAEs
- DBGMs
- SBGMs
- GANs
- Joint
- Applications
Contact
Location:
Amsterdam, the Netherlands
Email:
jmk.tomczakgmail.com